A year ago, Mark, the floor manager at a manufacturing company, would be up past midnight, buried in a sea of parts catalogs. He would be racing against time, trying to find the right parts for the assembly line that was set to start in just a few hours.
The old way of cross-referencing parts was slow, full of errors, and a big headache for everyone on the floor. But things have changed since then with the arrival of AI-enabled automation.
Now, instead of spending hours flipping through catalogs, Mark can find what he needs in minutes with the help of a digital tool that zips through the data with pinpoint accuracy. This change has been more than just a time-saver; it is a game-changer that promises a faster, smarter, and more reliable way of running the manufacturing floor.
With AI getting integrated into the process of of parts cross-referencing, the days of manual, error-prone processes are on their way out, making room for a new era of enhanced efficiency and precision in manufacturing.
A global survey revealed that AI decision-makers in manufacturing planning are gearing up to escalate their investments in AI, which indicates the technology’s growing importance in the manufacturing sector. Furthermore, a survey by KPMG underscored the rising eminence of AI in industrial manufacturing
While our previous blog, “Cutting Through Parts Cross-Referencing Clutter with AI-enabled Automation” laid down the foundational premise of AI’s potential in parts cross-referencing, it’s essential to move a step further. The journey from understanding the significance of AI to actual implementation in the manufacturing setup is laden with numerous considerations and steps. This progression needs a structured approach to unlock the full spectrum of benefits that AI automation promises.
This piece aims to navigate through this journey, providing a step-by-step guide aimed at assisting manufacturers in adopting AI automation for parts cross-referencing. The objective is to delineate a clear pathway, simplifying the transition towards an AI-enabled parts cross-referencing process.
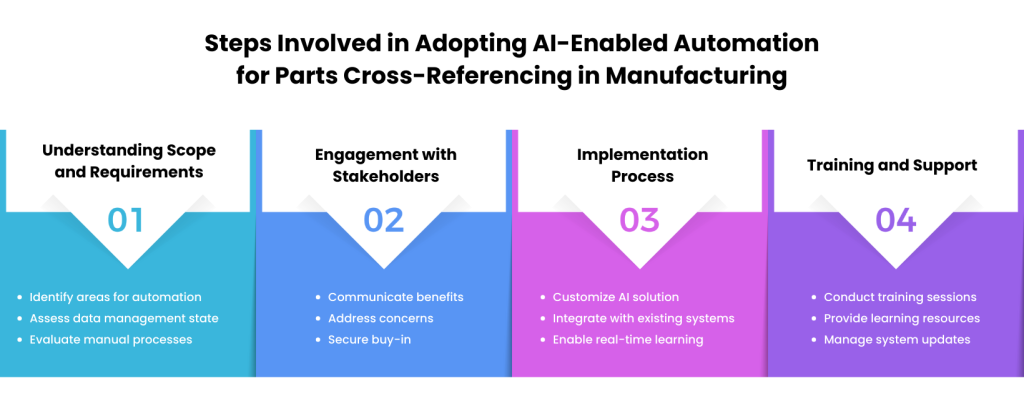
I. Understanding the Scope and Requirements
Automation of the parts cross-referencing process calls for a clear understanding of scope and a thorough analysis of the requisite requirements. A careful start in this stage sets the groundwork for a successful rollout, making sure it fits with company goals and gets the most out of AI-driven automation.
Here’s a closer look at the steps involved:
Identifying the Areas that Need Automation in Parts Cross-Referencing
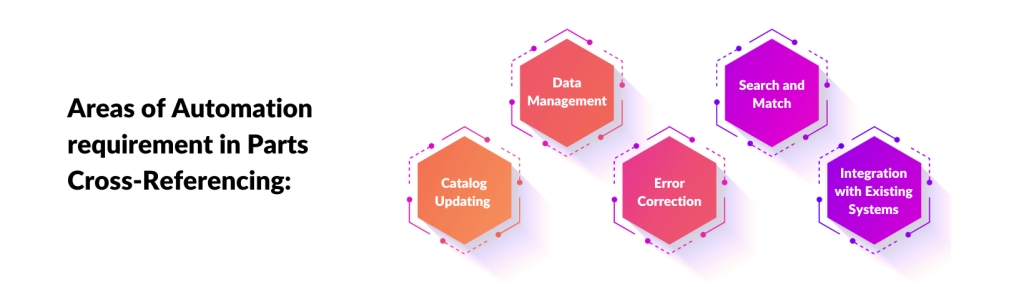
- Data Management:
Assess the current state of data management and identify areas where automation can streamline data collection, validation, and maintenance. Evaluate the potential of AI in handling diverse data formats and large datasets, which are intrinsic to parts cross-referencing.
- Search and Match:
Delve into the existing manual processes involved in searching and matching parts. Determine the intricacies and challenges that can be alleviated through automation. Explore how AI can enhance pattern recognition to identify similarities and variations among parts, making the matching process more accurate and efficient. - Catalog Updating:
Assess the manual efforts involved in keeping parts catalogs updated. Identify automated real-time updates, ensuring the catalogs reflect the most current information.
- Error Correction:
Identify the recurring errors in parts cross-referencing and understand how AI can automate the error correction process, reducing the scope of human errors.
- Integration with Existing Systems:
Determine how AI automation can seamlessly integrate with existing manufacturing software and databases, ensuring a smooth transition and operational coherence.
How can automation help in planning better
- Efficiency Optimization:
Define the goals related to enhancing operational efficiency, like reducing the time spent on parts cross-referencing and minimizing manual errors.
- Better Accuracy:
Set goals aimed at improving the accuracy of parts cross-referencing, ensuring the right parts are matched, which is crucial for maintaining the quality and functionality of the products. - Cost Reduction:
Establish goals pertaining to cost savings through reduced material wastage, minimized production downtime, and lower labor costs associated with manual cross-referencing. - Scalability:
Define the long-term goals of scaling the AI automation system as the operations grow and more products are introduced. This ensures the system remains efficient and effective.
- Competitive Edge:
Set forth the objectives related to gaining a competitive advantage in the market through quicker, accurate parts cross-referencing and staying ahead in embracing industry 4.0 technologies.
- ROI Realization:
Establish clear metrics to measure the return on investment (ROI) from automation adoption, creating a tangible gauge to assess the success of the implementation.
This preparatory stage is essential in ensuring that the subsequent steps in the AI adoption journey are well-informed and strategically sound, setting the stage for a successful transformation in parts cross-referencing operations.
II. Engaging with Stakeholders
Explain the Benefits and Address Concerns
- Communicating the benefits:
Clearly articulate the tangible benefits such as improved accuracy, efficiency, and cost savings that AI automation brings to parts cross-referencing. Highlight the strategic advantage and competitive edge it provides in the market, aligning with the broader business objectives.
- Concerns Addressal:
Actively listen to the concerns and questions of stakeholders, providing clear and precise answers. Assure them about the measures in place for a seamless transition and the training support for personnel to adapt to the new system.
Securing stakeholder buy-in is not merely about obtaining approval but building a collaborative environment where the key players are well-informed and invested in the initiative’s success. Their engagement and support are instrumental in navigating the implementation journey smoothly and realizing the envisioned benefits.
III. Implementation Process
Each facet of this process is designed to ensure that the adopted AI solution dovetails perfectly with existing processes, delivering the envisaged outcomes.
Customization and Configuration
- Tailoring the AI Automation Solution:
Collaborate with solution providers to tailor the AI-enabled automation to meet the distinct needs of parts cross-referencing within your manufacturing environment. Establish the rules, conditions, and parameters that the AI system will adhere to for precise cross-referencing of parts. - Configuring the System:
Engage in configuring the AI system to resonate with existing processes, data structures, and workflows, ensuring frictionless integration within the operational milieu. Validate that the system settings are primed to deliver precise and efficient cross-referencing results.
Integration
- Seamless Integration:
Pursue seamless integration of the AI system with prevailing manufacturing systems and databases, ensuring a continuum in operations. Forge robust data pipelines for real-time data interchange between the AI system and existing databases. Choose a solution that is platform agnostic to make the integration easy and effortless.
- Continuous Learning from Real-Time Data:
Enable the system for continuous learning from real-time data, nurturing an ongoing enhancement in accuracy and efficiency in cross-referencing. Keeping a vigilant eye on the system’s learning trajectory ensures it aligns with the set goals in automating cross-referencing.
This methodical approach to customization, integration, and training ascertains that the AI automation solution is well-aligned to significantly amplify the efficiency and accuracy of parts cross-referencing, driving the manufacturing operations towards a pinnacle of operational excellence.
IV. Training and Support
Navigating the realm of AI-enabled automation in parts cross-referencing necessitates a comprehensive understanding and adept handling of the system. It’s imperative to equip the workforce with the requisite knowledge and to ensure a robust support system is in place for an unhindered operational flow.
Employee Training
- Interactive Training Sessions:
Conduct structured training sessions enabling staff to proficiently interact with the new AI-enabled system. Utilize practical examples and real-time scenarios to explain the system’s functionalities and applications in parts cross-referencing. - Self-Learning and Troubleshooting Resources:
Provision a suite of resources fostering self-learning and troubleshooting. Offer manuals, online tutorials, and FAQ sections that employees can refer to for enhancing understanding and resolving common issues.
Ongoing Support
- Robust Support System:
Establish a steadfast support system to address technical issues promptly. Ensure a team of experts is accessible to provide guidance and resolve any system hitches that may impede the parts cross-referencing process. - System Updates and Upgrades:
Collaborate with the AI solution provider to receive timely updates and upgrades, keeping the system optimized and abreast with the latest advancements in AI technology. Ensure that each upgrade is followed by brief training sessions to acquaint the staff with new features and improvements.
This dual approach of comprehensive training and unwavering support not only smoothens the transition to an AI-enabled parts cross-referencing system but also underpins a culture of continuous learning and improvement. The objective is to foster a conducive environment where both the technology and the workforce evolve in tandem, driving towards a zenith of operational efficiency and accuracy in parts cross-referencing.
Conclusion
Heading into the realm of hyperautomation for parts cross-referencing isn’t just a leap towards better accuracy and efficiency in manufacturing, it’s a step into a broader, smarter way of operating.
This shift, supported by powerful AI-driven automation solutions, showcases the limitless possibilities of AI in reshaping the way we work. It’s more than just speeding up a process; it’s about lifting the whole operational game to a level where smart, data-backed decisions are the norm.
The core of this effort goes beyond just parts cross-referencing, urging manufacturers to fully welcome the era of AI-first hyperautomation. It’s a call to step into a future where achieving operational excellence is an ongoing journey, not just a distant goal.